At MediaX, we’re constantly experimenting with scalable and generalizable AI models. One of our latest research efforts involved leveraging the GLASS framework—Generalizable Local Anomaly Segmentor—to detect and segment visual anomalies in image datasets. Our evaluation demonstrates highly promising performance for real-world applications.
Framework
GLASS is a state-of-the-art deep learning model tailored for anomaly segmentation. It combines a powerful encoder-based architecture with local feature learning to detect subtle defects and anomalies in complex backgrounds.
We trained the model on our internal MediaX Encoder Dataset, using Colab and custom shell scripts for execution and result export. The model training and evaluation were done using GLASS’s native runner, integrated with our customized data pipeline.
Performance Highlights
Image-Level Detection
- AUROC: 100.0
- Average Precision (AP): 100.0
This indicates perfect classification between normal and anomalous images.
Pixel-Level Localization
- AUROC: 96.99
- Average Precision: 19.19
- PRO (Per-Region Overlap): 95.03
While the model localizes anomaly regions very effectively (high AUROC/PRO), the lower AP suggests slight noise sensitivity or over-segmentation—an area we are actively improving.
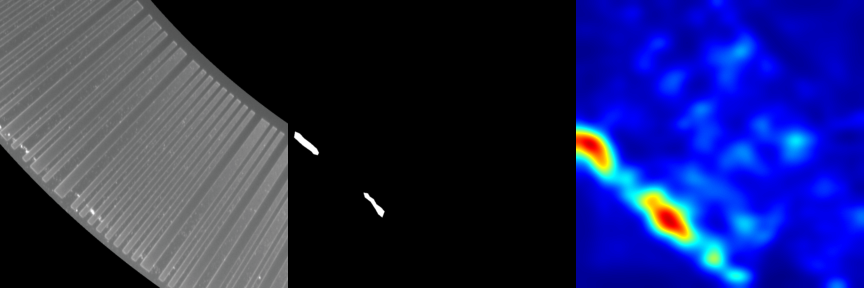
Impact and Next Steps
This research validates the effectiveness of GLASS for industry-level visual inspection. We aim to further optimize pixel-level precision and apply the framework across broader verticals like manufacturing, logistics, and smart surveillance.
To follow our latest AI research, visit the MediaX Research Page.